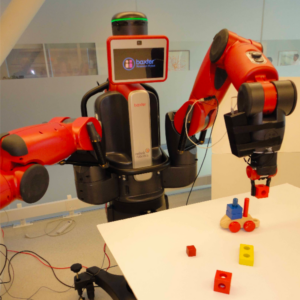
Babies develop the abilities to perceive and act without being taught how to do so. We would like to accomplish the same in a mobile robot equipped with cameras, microphones, tactile skins, and manipulators. We launch such a robot and a blank-slate machine learning system with minimal built-in reflexes and assumptions. By exerting actions and interacting with the world, the robot collects sensor-motor data. By studying the regularity and structures contained in such data, the robot learns to control its own body, then to have deliberate effects on certain objects that it can recognize. Over time it learns a hierarchy of increasingly complex actions, and an increasing depth of knowledge about the objects it encounters and the affordances they provide. Such a system can adapt easily to not only its environment but also its own body, requiring no knowledge of the 3D external world or its physical body. Such computational work could shed light on the development of intelligence of an embodied agent without any supervision.
People
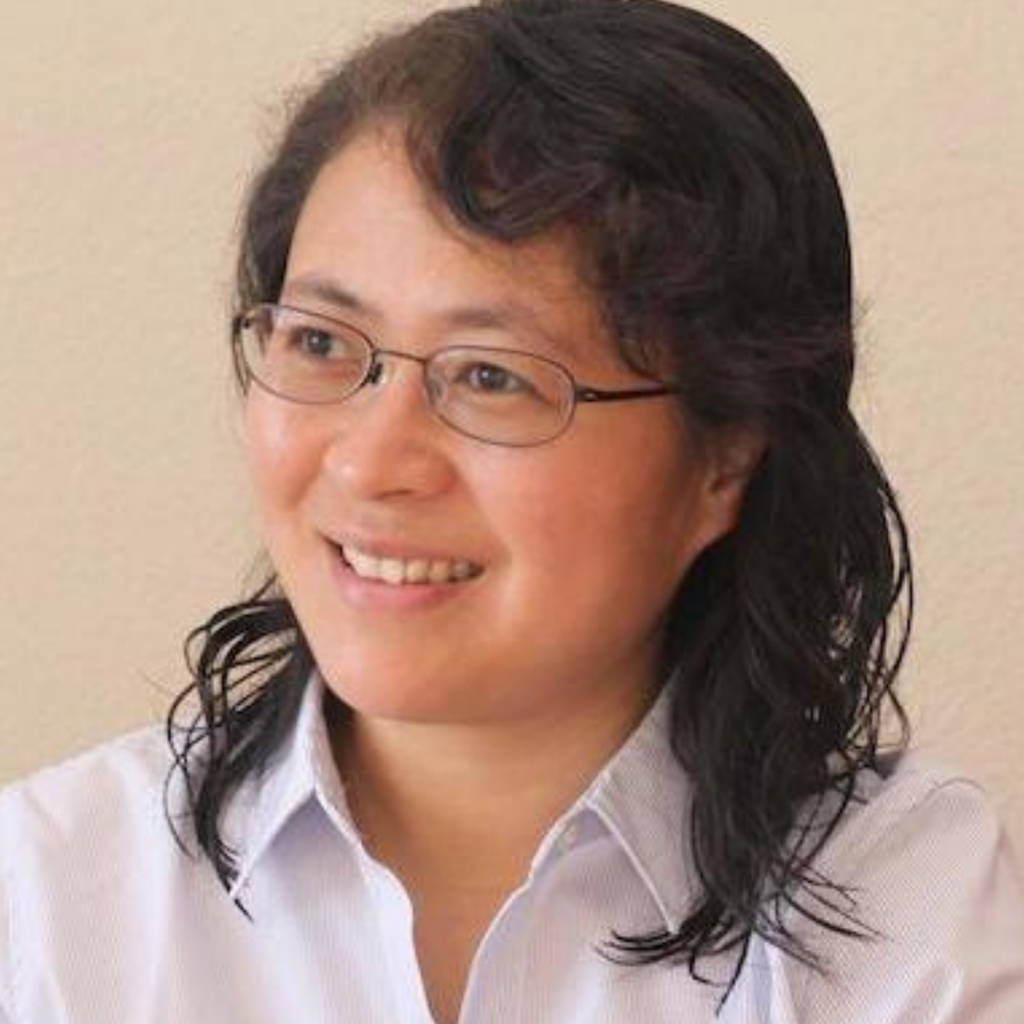
Stella
Yu
CSE
Engineering
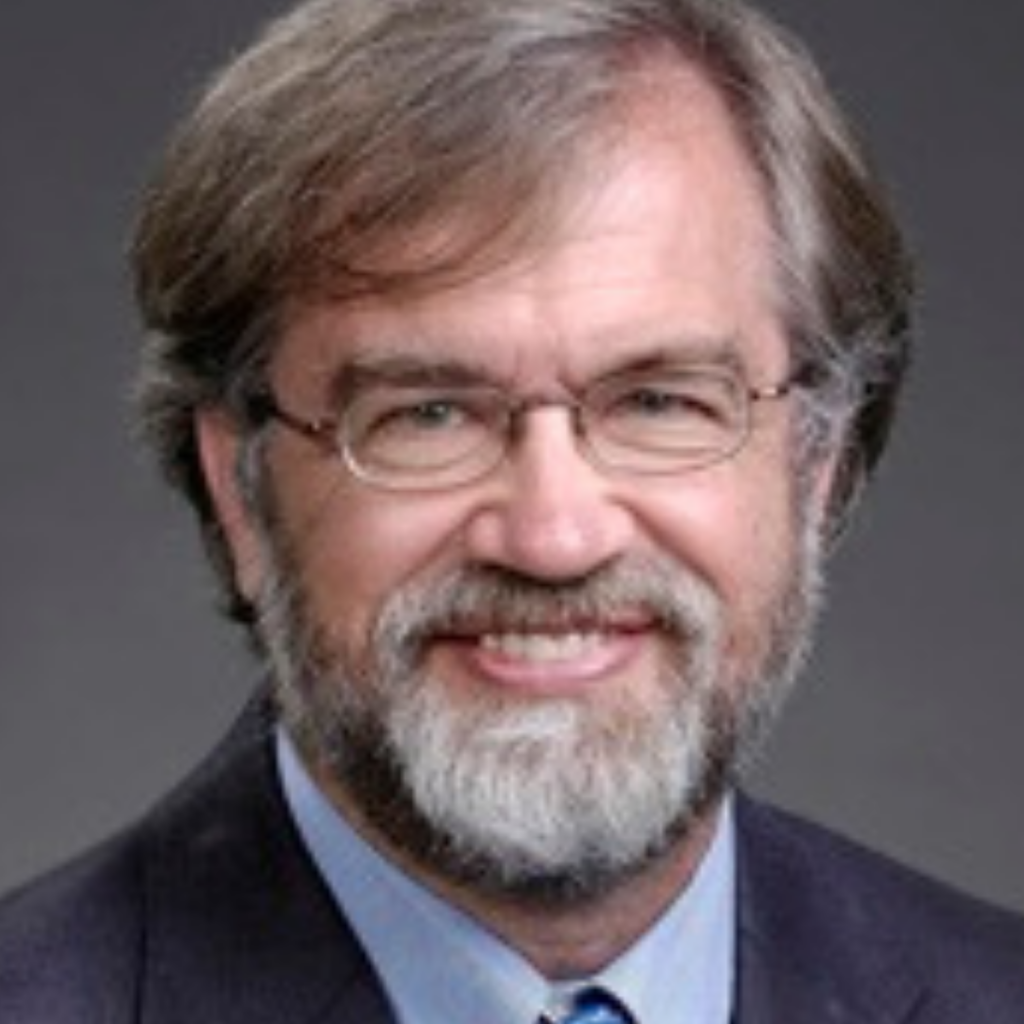
Ben
Kuipers
CSE
Engineering
Funding
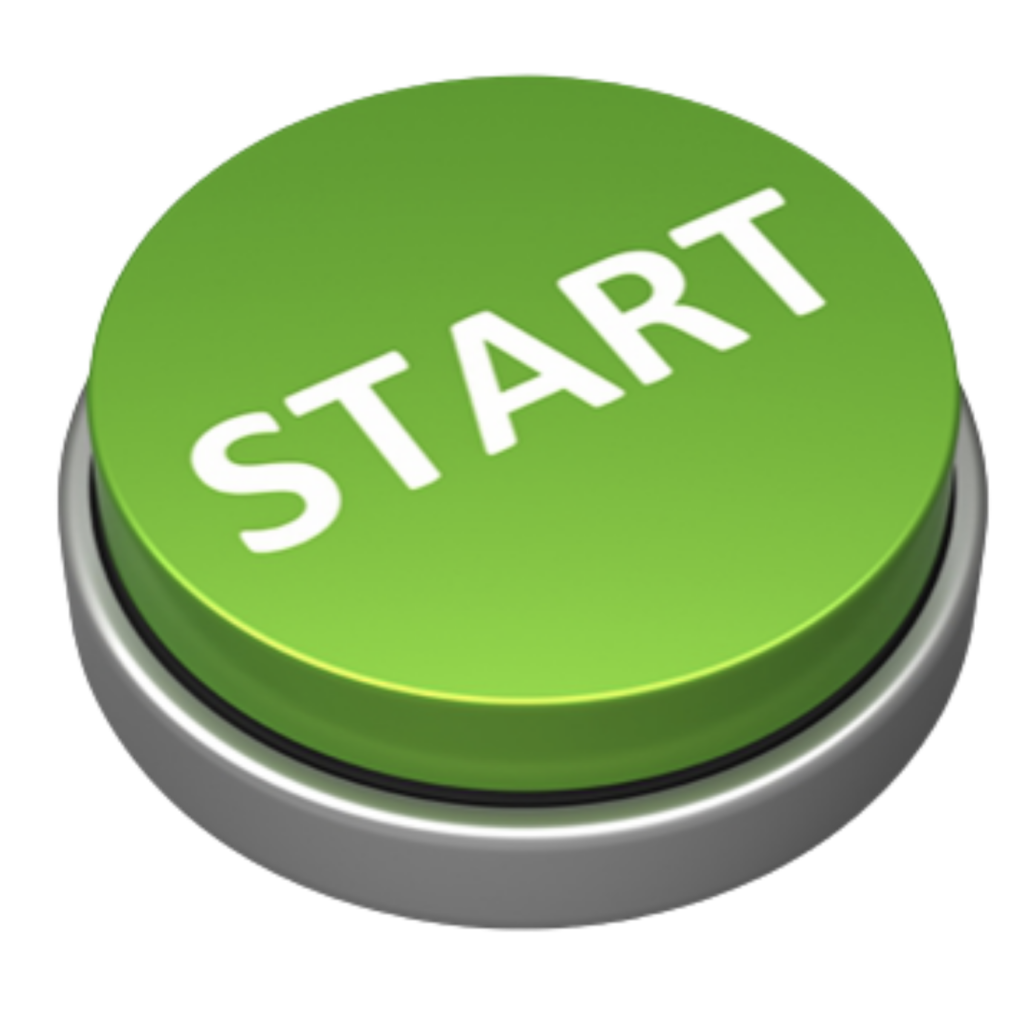
Funding: $30K (2023)
Goal: Our goal is to develop a robot that can learn how to act and perceive automatically from its interactions with the world.
Token Investors: Stella Yu and Ben Kuipers
Project ID: 1119