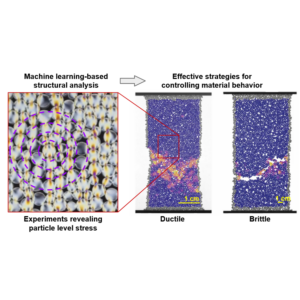
The deformation behavior of amorphous materials like granular materials, colloids, biological tissues, and metallic glasses is hard to control due to the lack of understanding for how their local disordered packing structure interacts with dynamics. Recent studies have shown that effective structural descriptors can be developed using machine-learning based methods, which can be related to local mechanical properties to enable physics-based modeling. Here, we aim to integrate this methodology into material preparation to achieve controllable deformation behaviors. More specifically, we will develop a machine learning agent to assess instantaneous structure and update preparation processes such as annealing, mechanical aging, and defect planting. Such an idea will be tested in simulations of particle systems as well as lab experiments using customized granular particles. We will measure the local packing structure, stresses, and dynamics to inform the machine learning agent. The physics that connects the microscopics and macroscopic material behaviors such as shear banding and fracture will also be probed.
People
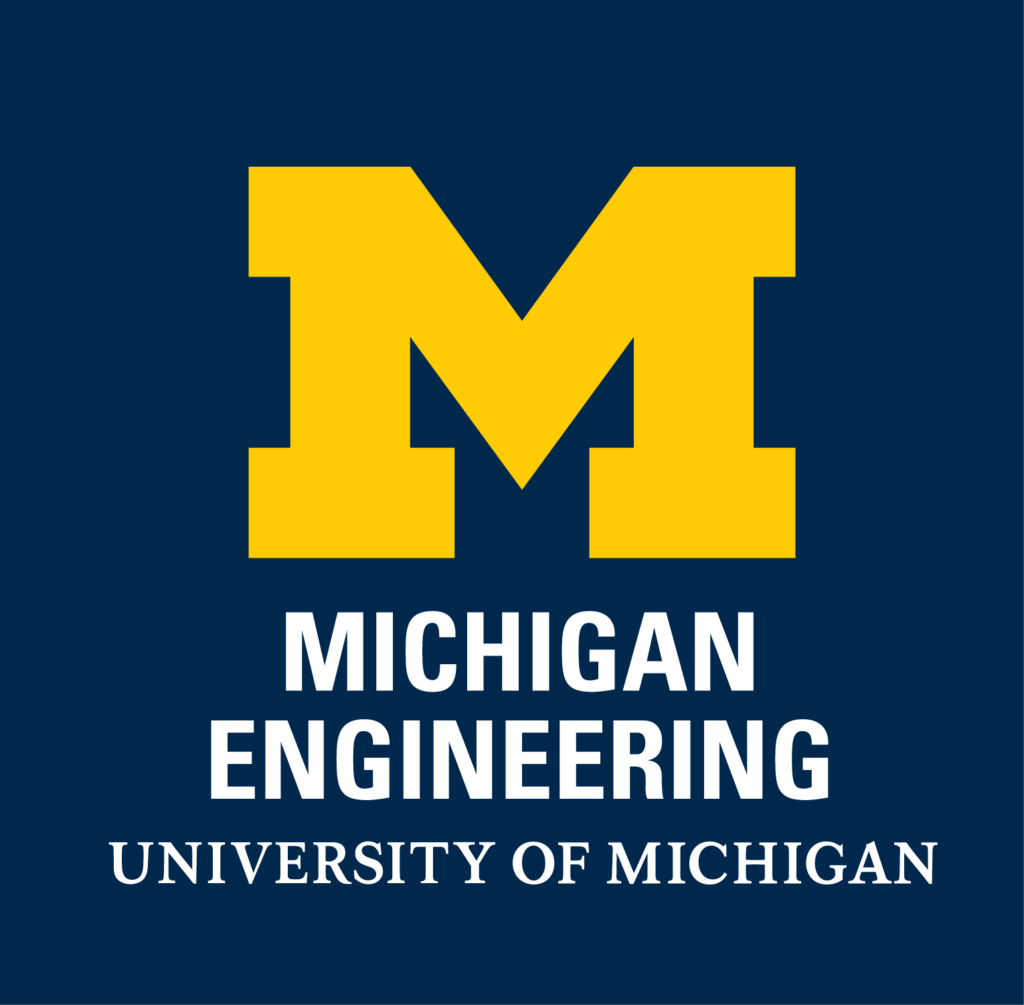
Hongyi
Xiao
ME
Engineering
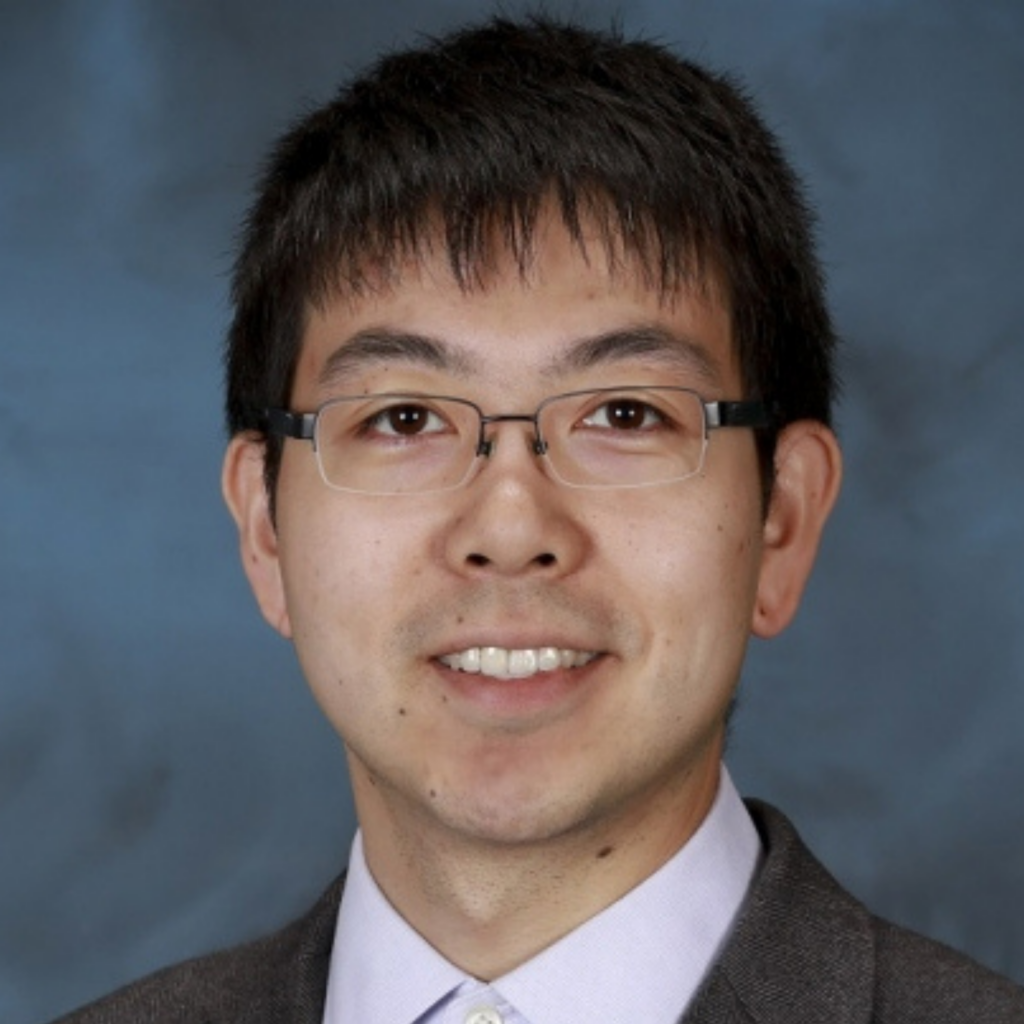
Yue
Fan
ME
Engineering
Funding
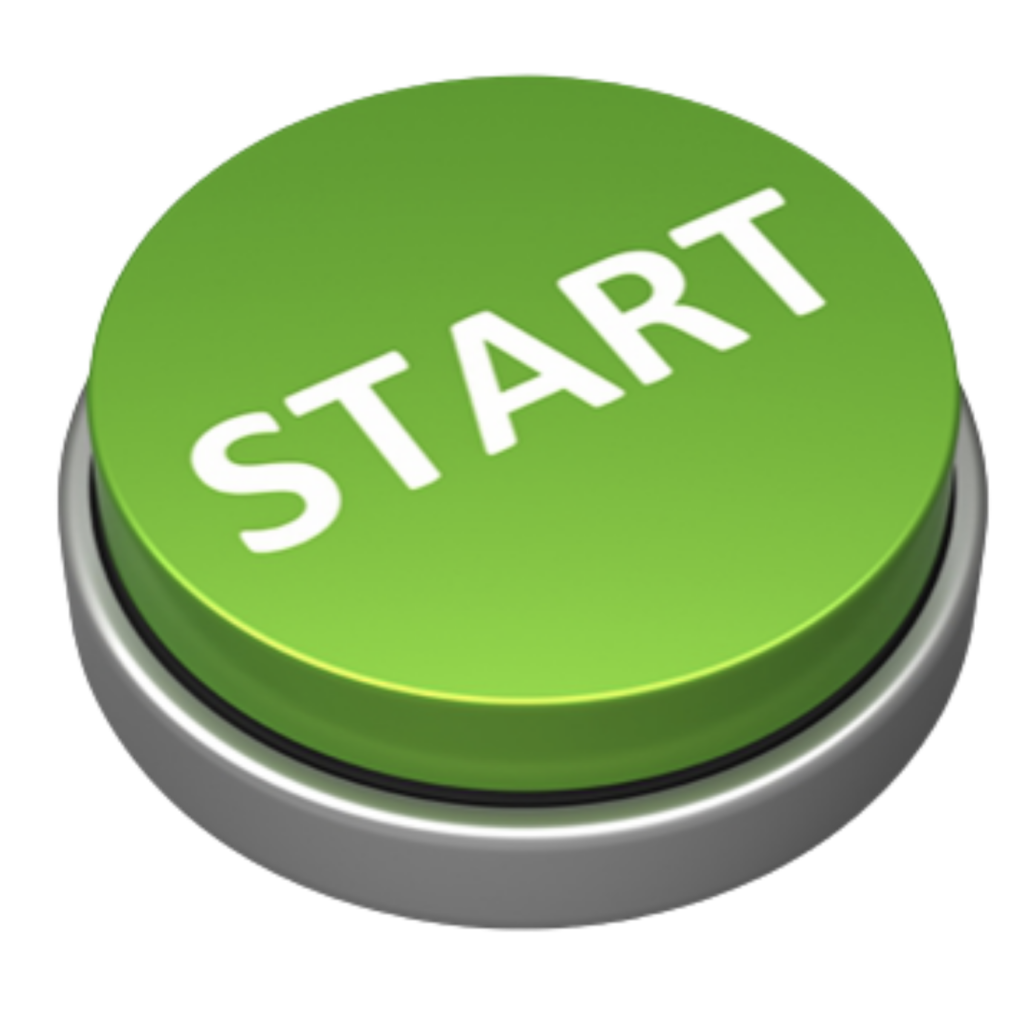
Funding: $30K (2023)
Goal: Uncover the essential physics needed for enabling machine learning-informed preparation for amorphous materials with desired deformation behavior
Token Investors: Hongyi Xiao and Yue Fan
Project ID: 1115