Reliable, physically transparent, and predictive models that can accurately quantify chemical and physical interactions between a surface of a material and adsorbate molecules are important in many fields of chemistry, physics, and material science. Although there are theoretical models that can at least qualitatively capture molecular adsorption on metal surfaces, the adsorption trends on single atom alloys (SAAs) are less well understood. Single-atom alloys (SAAs) are an emerging class of single-site catalysts in which small amounts of isolated metal atoms are present in the surface layer of a metal host. We propose to use interpretable machine learning approaches to develop meaningful descriptors of molecule adsorption and reactivity on SAAs and therefore unearth fundamental structure-activity relationships that will guide the discovery of SAA catalysts for important catalytic reactions.
People
Funding
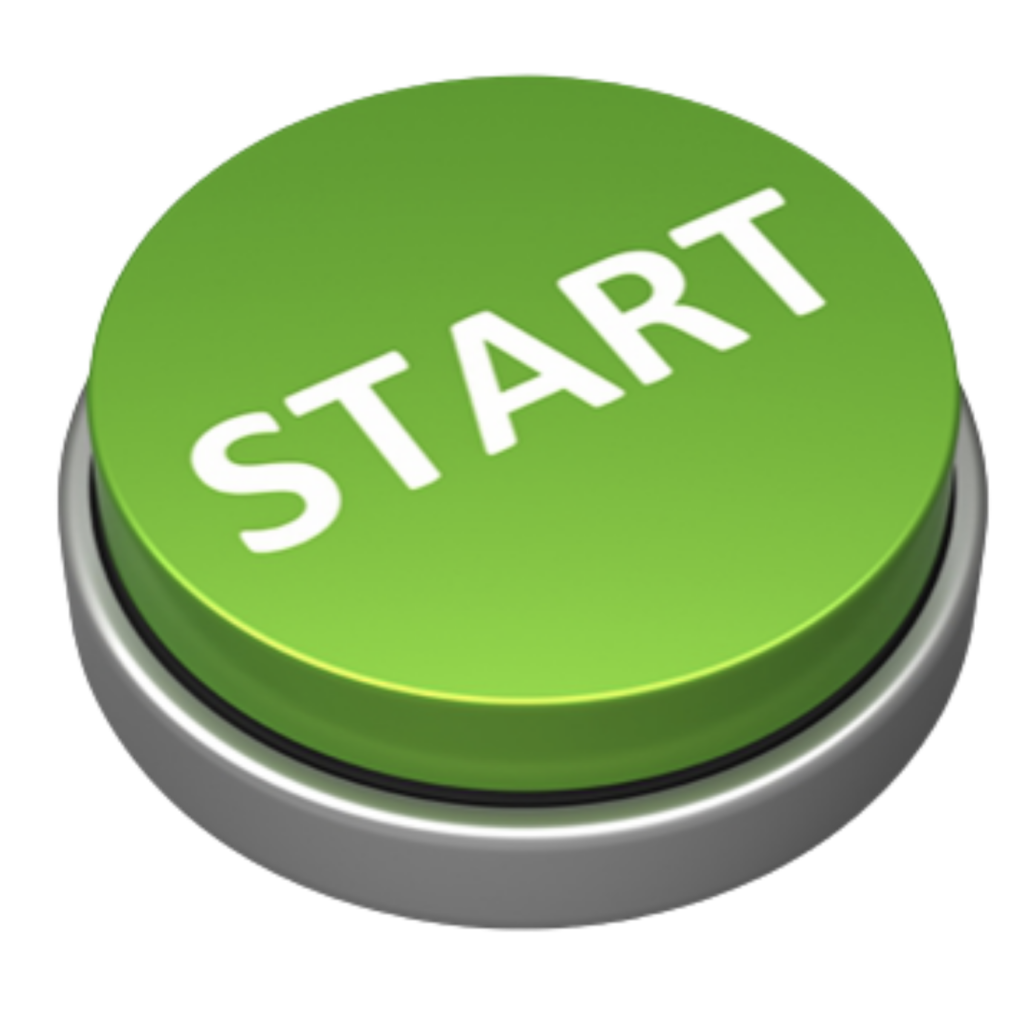
Funding: $30K (2022)
Goal: Our goal is to develop interpretable machine learning workflows to shed light on the critical features of single-atom alloys that govern the adsorption of adsorbates and provide links to catalyst stability and activity.
Token Investors: Bryan Goldsmith, Suljo Linic
Project ID: 2023